Edition: August 22nd, 2021
Curated by the Knowledge Team of ICS Career GPS
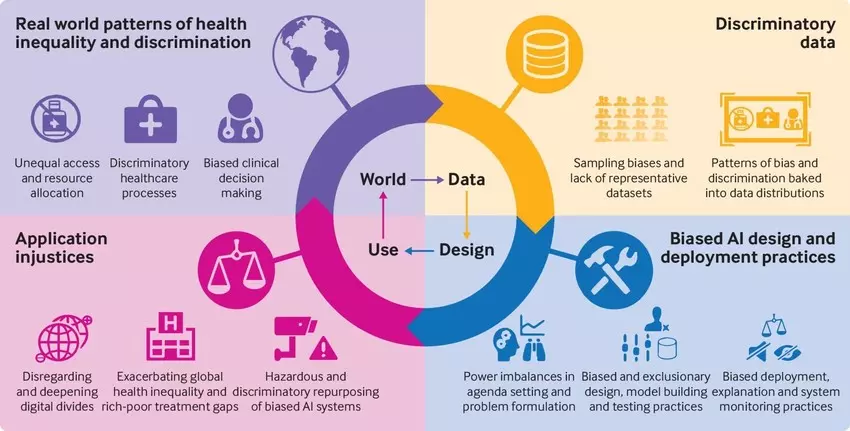
- Excerpts from article by Agbolade Omowole, published on World Economic Forum
Can you imagine a just and equitable world where everyone, regardless of age, gender or class, has access to excellent healthcare, nutritious food and other basic human needs?
Are data-driven technologies such as artificial intelligence and data science capable of achieving this – or will the bias that already drives real-world outcomes eventually overtake the digital world, too?
Bias represents injustice against a person or a group. A lot of existing human bias can be transferred to machines because technologies are not neutral; they are only as good or bad as the people who develop them.
Here are the different types of biases that can creep into AI systems:
1. Implicit Bias
Implicit bias is discrimination or prejudice against a person or group that is unknown to the person with the bias. It is dangerous because the person is unaware of the bias – whether it be on grounds of gender, race, disability, sexuality or class. The person is unconsciously propagating it.
2. Sampling Bias
This is a statistical problem where random data sets selected from the population do not reflect the distribution of the population. The sample data may be skewed towards some subset of the group.
3. Temporal Bias
This is based on our perception of time. We can build a machine-learning model that works well at this time, but fails in the future because we didn’t factor in possible future changes when building the model.
4. Over-fitting to Training Data
This happens when the AI model can accurately predict values from the training dataset but cannot predict new data accurately. The model adheres too much to the training dataset and does not generalise to a larger population.
5. Edge Cases & Outliers
These are data outside the boundaries of the training dataset. Outliers are data points outside the normal distribution of the data. Errors and noise are classified as edge cases.
- Errors are missing or incorrect values in the dataset
- Noise is data that negatively impacts the machine learning process
How to identify & correct these biases?
⦿ Analytical techniques
- Analytical techniques require meticulous assessment of the training data for sampling bias and unequal representations of groups.
- You can investigate the source and characteristics of the dataset. Check the data for balance.
- Without strong analytical study of the data, the cause of the bias will be undetected and unknown.
⦿ Test the model in different environments
- Expose your model to varying environments and contexts for new insights.
- You want to be sure that your model can generalise to a wider set of scenarios.
- The problem with some algorithms’ development is that they are not tested properly before deployment.
⦿ Inclusive design and foreseeability
- Inclusive design emphasises inclusion in the design process.
- The AI product should be designed with consideration for diverse groups such as gender, race, class and culture.
- Foreseeability is about predicting the impact the AI system will have right now and over time.
⦿ Perform user testing
- Testing is an important part of building a new product or service.
- User testing in this case refers to getting representatives from the diverse groups that will be using your AI product to test it before it is released.
⦿ STEEPV analysis
- This is a method of performing strategic analysis of external environments. It is an acronym for social, technological, economic, environmental, political and values.
- Performing a STEEPV analysis will help you detect fairness and non-discrimination risks in practice.
Tacking inequality
- The COVID-19 pandemic and recent social and political unrest have created a profound sense of urgency for companies to actively work to tackle inequity.
- It is very easy for the existing bias in our society to be transferred to algorithms.
- There is an urgent need for corporate organisations to be more proactive in ensuring fairness and non-discrimination as they leverage AI to improve productivity and performance.
One possible solution is by having an AI ethicist in your development team to detect and mitigate ethical risks early in your project before investing lots of time and money.
…
(Disclaimer: The opinions expressed in the article mentioned above are those of the author(s). They do not purport to reflect the opinions or views of ICS Career GPS or its staff.)